Supported by |
|
|
Additional cooperation from |
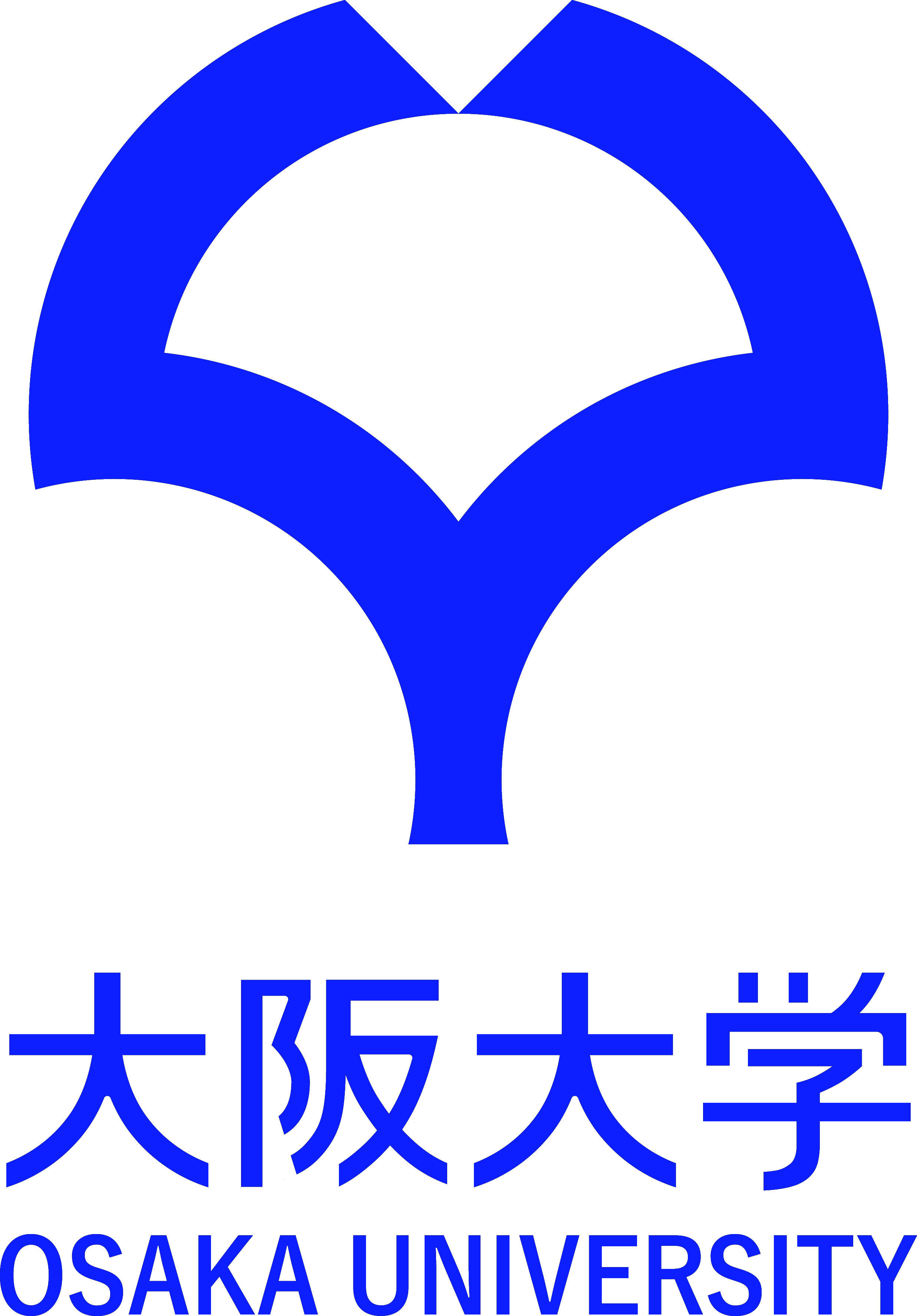
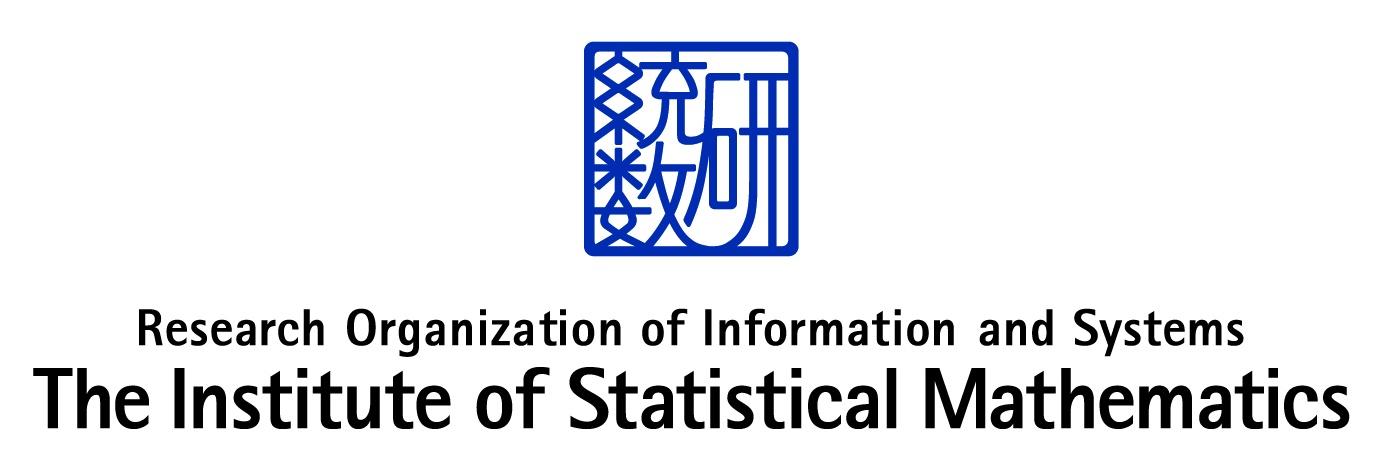 |
|
Third International Workshop on Symbolic-Neural Learning (SNL-2019)
July 11-12, 2019 Miraikan hall, Odaiba Miraikan 7F (Tokyo, Japan)
Symbolic-neural learning involves deep learning methods in combination with symbolic structures. A "deep learning method" is taken to be a learning process based on gradient descent on real-valued model parameters. A "symbolic structure" is a data structure involving symbols drawn from a large vocabulary; for example, sentences of natural language, parse trees over such sentences, databases (with entities viewed as symbols), and the symbolic expressions of mathematical logic or computer programs. Natural applications of symbolic-neural learning include, but are not limited to, the following areas:
- Image caption generation and visual question answering
- Speech and natural language interactions in robotics
- Machine translation
- General knowledge question answering
- Reading comprehension
- Textual entailment
- Dialogue systems
Various architectural ideas are shared by deep learning systems across these areas. These include word and phrase embeddings, recurrent neural networks (LSTMs and GRUs) and various attention and memory mechanisms. Certain linguistic and semantic resources may also be relevant across these applications. For example dictionaries, thesauri, WordNet, FrameNet, FreeBase, DBPedia, parsers, named entity recognizers, coreference systems, knowledge graphs and encyclopedias. Deep learning approaches to the above application areas, with architectures and tools subjected to quantitative evaluation, loosely define the focus of the workshop.
The workshop consists of invited oral presentations and contributed poster presentations.
Organizing Committee:
Jun'ichi Tsujii (Chair) |
AIST AI Research Center, Tokyo, Japan and
the University of Manchester, Manchester, UK |
Sadaoki Furui |
Toyota Technological Institute at Chicago, Chicago, USA |
Tomoko Matsui |
The Institute of Statistical Mathematics, Tokyo, Japan |
David McAllester |
Toyota Technological Institute at Chicago, Chicago, USA |
Yutaka Sasaki |
Toyota Technological Institute, Nagoya, Japan |
Koichi Shinoda |
Tokyo Institute of Technology, Tokyo, Japan |
Masashi Sugiyama |
RIKEN Center for AIP and the University of Tokyo, Tokyo, Japan |
Hiroya Takamura |
AIST AI Research Center and Tokyo Institute of Technology, Tokyo, Japan |
Yasushi Yagi |
Osaka University, Osaka, Japan |
Program Committee:
Hiroya Takamura (Chair) |
AIST AI Research Center and Tokyo Institute of Technology, Tokyo, Japan |
Yuki Arase |
Osaka University, Osaka, Japan |
Nakamasa Inoue |
Tokyo Institute of Technology, Tokyo, Japan |
Kentaro Inui |
RIKEN Center for AIP and Tohoku University, Sendai, Japan |
Makoto Miwa |
Toyota Technological Institute, Nagoya, Japan |
Daichi Mochihashi |
The Institute of Statistical Mathematics, Japan |
Greg Shakhnarovich |
Toyota Technological Institute at Chicago, Chicago, USA |
Previous Workshops:
|