Eighth International Workshop on Symbolic-Neural Learning (SNL2024)
June 26-27, 2024
Miraikan hall, Odaiba Miraikan 7F (Tokyo, Japan)
Keynote Talks:
Marco Cuturi (Apple ML Research)
"A Short Survey on Optimal Transport Problems Arising in Sciences and Machine Learning and their Numerical Resolution using Neural Networks"
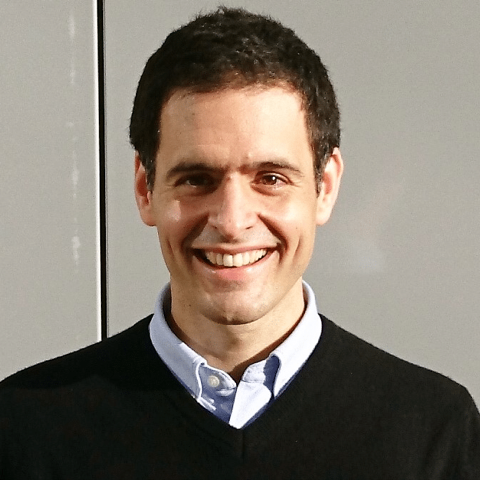
Abstract:
Given samples from a source and a target distribution, many problems arising in natural sciences and machine learning involve inferring a "least-effort" transformation to re-map these source points to target points. This problem arises for instance in personalised medecine, when trying to predict the effect of a cancer treatment by only relying on two unaligned point cloud descriptions of cells, sampled before and after applying a treatment. This problem is also prominently featured in generative modelling, which is concerned with re-creating objects (e.g. images) from noise, and possibly conditioned on extra labels (e.g. text). The optimal transport problem was originally formulated by Gaspard Monge in the late 18th century, and has experienced, in the span of 2 decades, dramatic developments, both in mathematics, computations, statistics and machine learning. After giving a brief introduction to this problem, I will describe a few recent computational approaches that use insights from mathematics to improve the quality of OT estimators.
Bio:
Marco Cuturi is a researcher at Apple's Machine Learning Research group, led by Samy Bengio, since 2022. His work focuses on the computations of optimal transport and their application to machine learning and natural sciences. MC is also a professor at ENSAE, IP Paris, now part-time, since 2016. He was a member of the Google Brain team between 2018 and 2022, and was a tenured associate professor in the Graduate School of Informatics, Kyoto University, between 2010 and 2016. Before that he was a lecturer at Princeton University (ORFE, 2009~2010), an associate in a hedge fund at crédit suisse (2007~2008), and a post-doctoral researcher at the Institute of Statistical Mathematics (2005~2006). He received his Ph.D. in 11/2005 from Ecole des Mines de Paris.
Graham Neubig(Carnegie Mellon University)
"What is the shortest path to generalist web agents?"
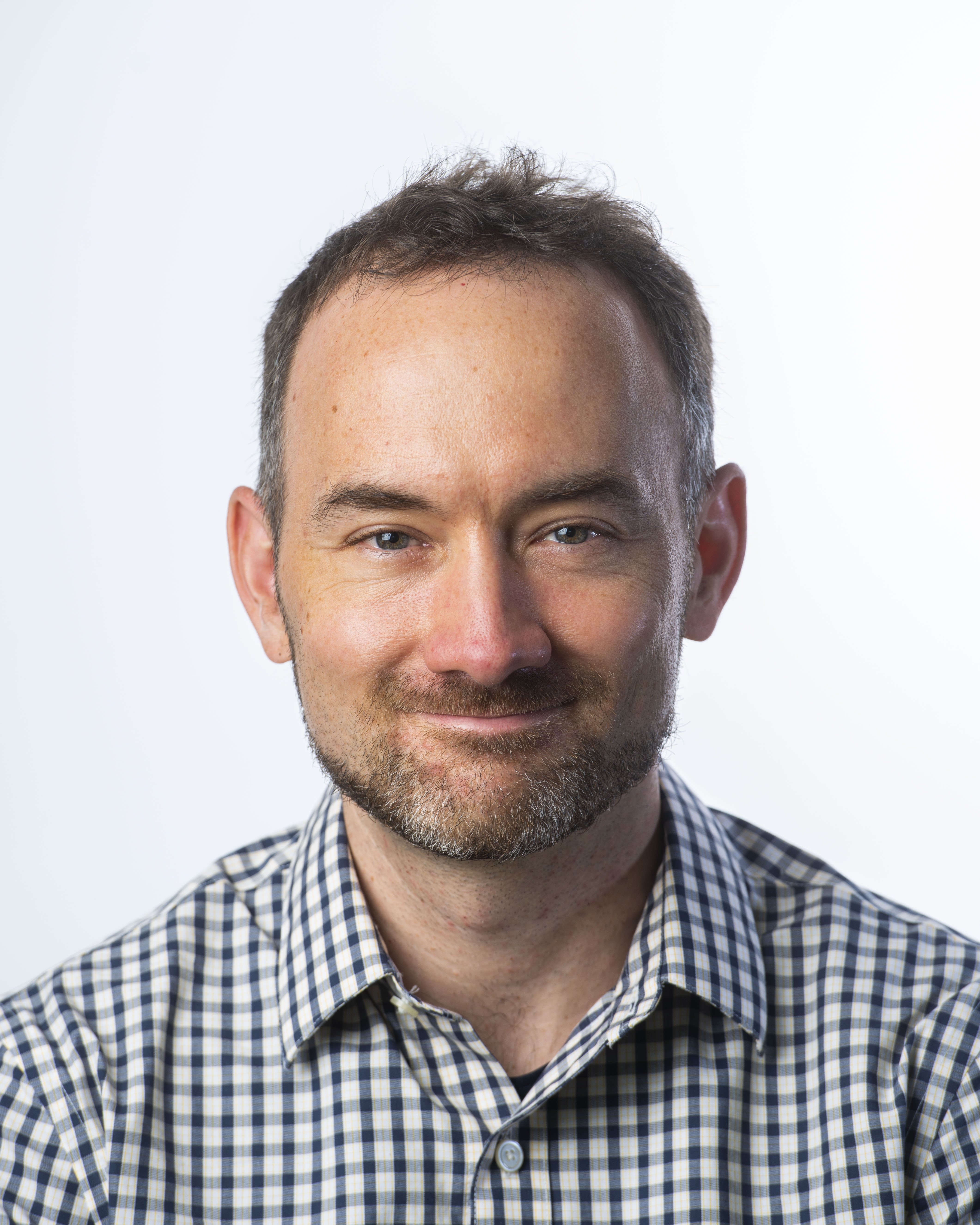
Abstract:
The research world is abuzz with talk of AI agents that are built on a backbone of large language models (LLMs) imbued with the ability to call external APIs or tools that allow them to act in the world. However, at the moment these generalist agents are still largely a curiosity, there are few workflows in our life where we can replace our own actions directly with those of an AI agent. What progress have we made in general AI agents, and what are the challenges that are holding us back? In this talk I will talk about four major challenges in building these AI agents, some work at CMU that we are performing that starts to tackle these problems, and a future roadmap towards generalist AI:
1. Realistic evaluation in consequential settings
2. Language models that can plan and execute long trajectories
3. Understanding of complex real-world textual, visual, and data-grounded environments
4. Learning from human feedback -- imitation and reinforcement learning
Bio:
Graham Neubig is an associate professor at the Language Technologies Institute of Carnegie Mellon University. His research focuses on natural language processing, with a particular interest in fundamentals, applications, and understanding of large language models for tasks such as question answering, code generation, and multilingual applications. His final goal is that every person in the world should be able to communicate with each-other, and with computers in their own language. He also contributes to making NLP research more accessible through open publishing of research papers, advanced NLP course materials and video lectures, and open-source software, all of which are available on his web site.
Boxin Shi (Peking University)
High-speed Photometric Analysis using the Neuromorphic Camera
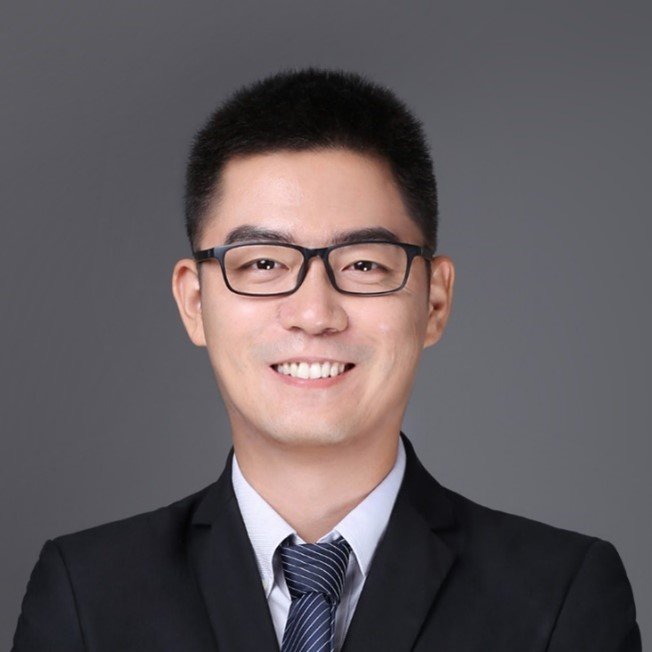
Abstract:
Compared with conventional frame-based cameras, the neuromorphic cameras, such as the event camera and the spike camera have unique advantages, especially in their ability to perceive high-speed moving objects and scenes with high dynamic range. Existing research has actively demonstrated the advantages of event cameras in computer vision tasks such as image deblurring, high dynamic range imaging, and high-speed object detection and recognition. However, the photometric image formation model of event cameras has not been carefully analyzed. This talk will share a series of research progress on modeling and analyzing the photometric image formation model of an event camera that records and responses to high-speed radiance changes: obtaining high-fidelity scene radiance estimation via analyzing the transient event frequency, conducting direct-global illumination separation by capturing shadows of a line occlude swiftly sweeping over the scene, and constructing real-time photometric stereo with fast-moving light sources to estimate surface normal.
Bio:
Boxin Shi is currently a Boya Young Fellow Associate Professor (with tenure) and Research Professor at Peking University, where he leads the Camera Intelligence Lab. He has also been a Young Scientist at Beijing Academy of Artificial Intelligence. He received the PhD degree from the University of Tokyo in 2013. From 2013 to 2017, he did research at MIT Media Lab, Singapore University of Technology and Design, Nanyang Technological University, and National Institute of Advanced Industrial Science and Technology. His research interests are computational photography and computer vision. He has published more than 200 papers, including 25 papers in TPAMI and 82 papers in CVPR/ICCV/ECCV. His papers were awarded as Best Paper - Runner Up at International Conference on Computational Photography 2015 and selected as Best Papers from ICCV 2015 for IJCV Special Issue. He received the Okawa Foundation Research Grant in 2021. He has served as an associate editor of TAPMI/IJCV and an area chair of CVPR/ICCV/ECCV. He is a Senior Member of the IEEE/CCF/CSIG, and a Distinguished Lecturer of APSIPA. Please access his lab website for more information: http://camera.pku.edu.cn
Emre Ugur (Bogazici University)
DeepSym: A Neuro-symbolic Approach for Symbol Emergence and Planning
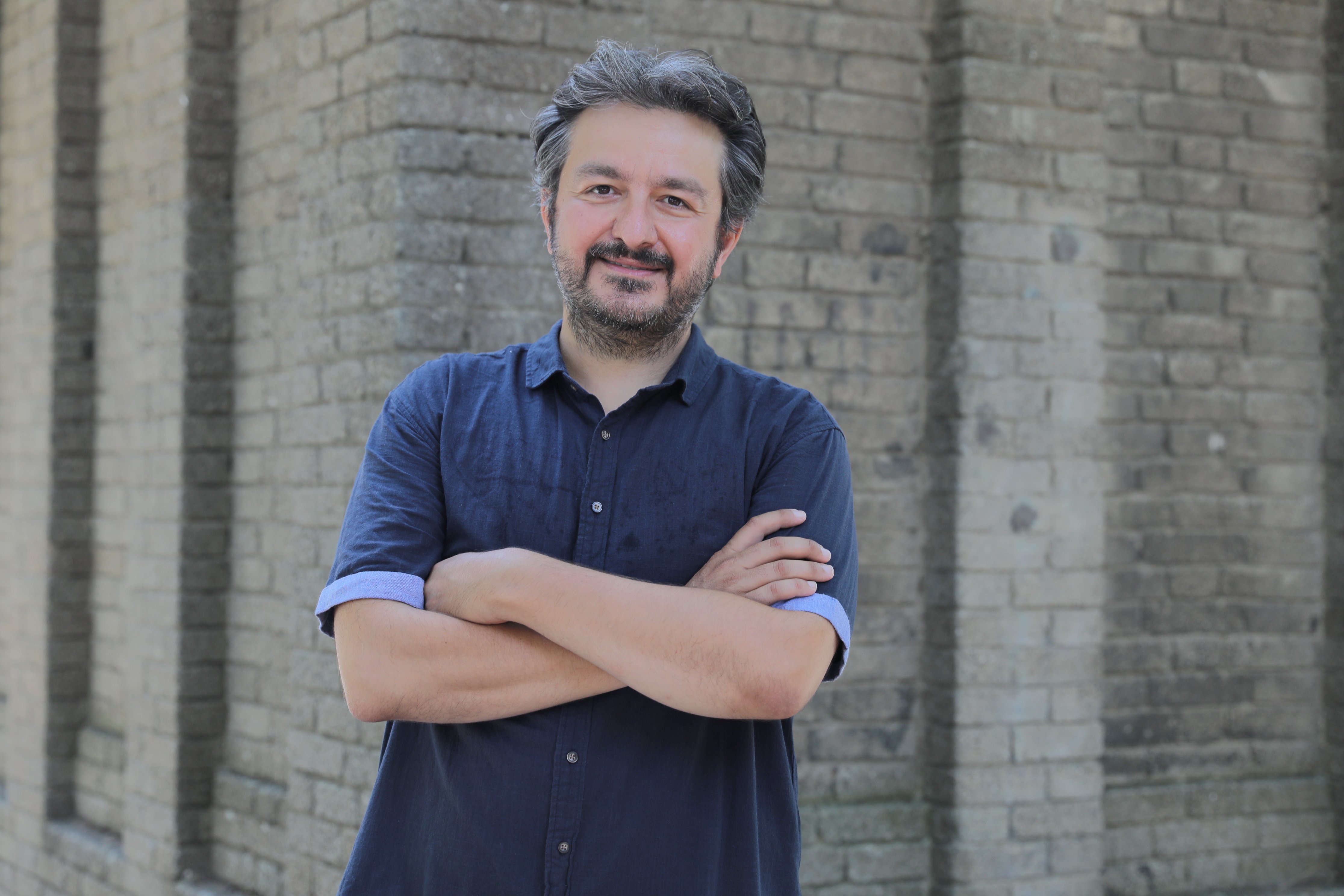
Abstract:
Abstraction and abstract reasoning are among the most essential
characteristics of high-level intelligence that distinguish humans
from other animals. High-level cognitive skills can only be achieved
through abstract concepts, symbols representing these concepts, and
rules that express relationships between symbols. If the robots can
achieve abstract reasoning on their own, they can perform new tasks in
completely novel environments by updating their cognitive skills or by
discovering new symbols and rules. Towards this goal, we propose a
novel general framework, DeepSym, which discovers action-grounded,
discrete object and effect categories and builds probabilistic rules
for non-trivial action planning. If the objectives of this endeavor
are achieved, scientific foundations will be laid for robotic systems
that learn life-long lasting symbols and rules through
self-interacting with the environment and express various
sensory-motor and cognitive tasks in a single framework.
In DeepSym, our robot interacts with objects using an initial action
repertoire and observes the effects it can create in the environment.
To form action-grounded object, effect, and relational categories, we
employ a binary bottleneck layer in a predictive, deep encoder-decoder
network that takes the image of the scene and the action applied as
input and generates the resulting effects in the scene in pixel
coordinates. The knowledge represented by the neural network is
distilled into rules and represented in the Probabilistic Planning
Domain Definition Language (PPDDL), allowing off-the-shelf planners to
operate on the knowledge extracted from the sensorimotor experience of
the robot. I will also present how this architecture can be extended
to learn symbols for compounds of varying numbers of objects using
Graph Neural Networks and Attention layers.
Bio:
Emre Ugur is an Associate Professor in Dept. of Computer Engineering
at Bogazici University, the chair of the Cognitive Science
MA Program, and the head of the Cognition, Learning, and Robotics
(CoLoRs) lab. He received his Ph.D. degree in Computer Engineering
from Middle East Technical University (METU, Turkey). He was a
research assistant at METU (2003-2009), worked as a research scientist
at ATR, Japan (2009-2013), and visited Osaka University as a specially
appointed Assoc. Professor (2016); and worked as a senior researcher
at the University of Innsbruck (2013-2016). He has been awarded The
Young Scientist Award by the Science Academy (BAGEP) and The
Excellence in Teaching Award by the Faculty of Engineering in 2023. He
is interested in robotics, robot learning, and cognitive robotics.
Shinji Watanabe (Carnegie Mellon University)
"Reproducing Large Speech Foundation Models"
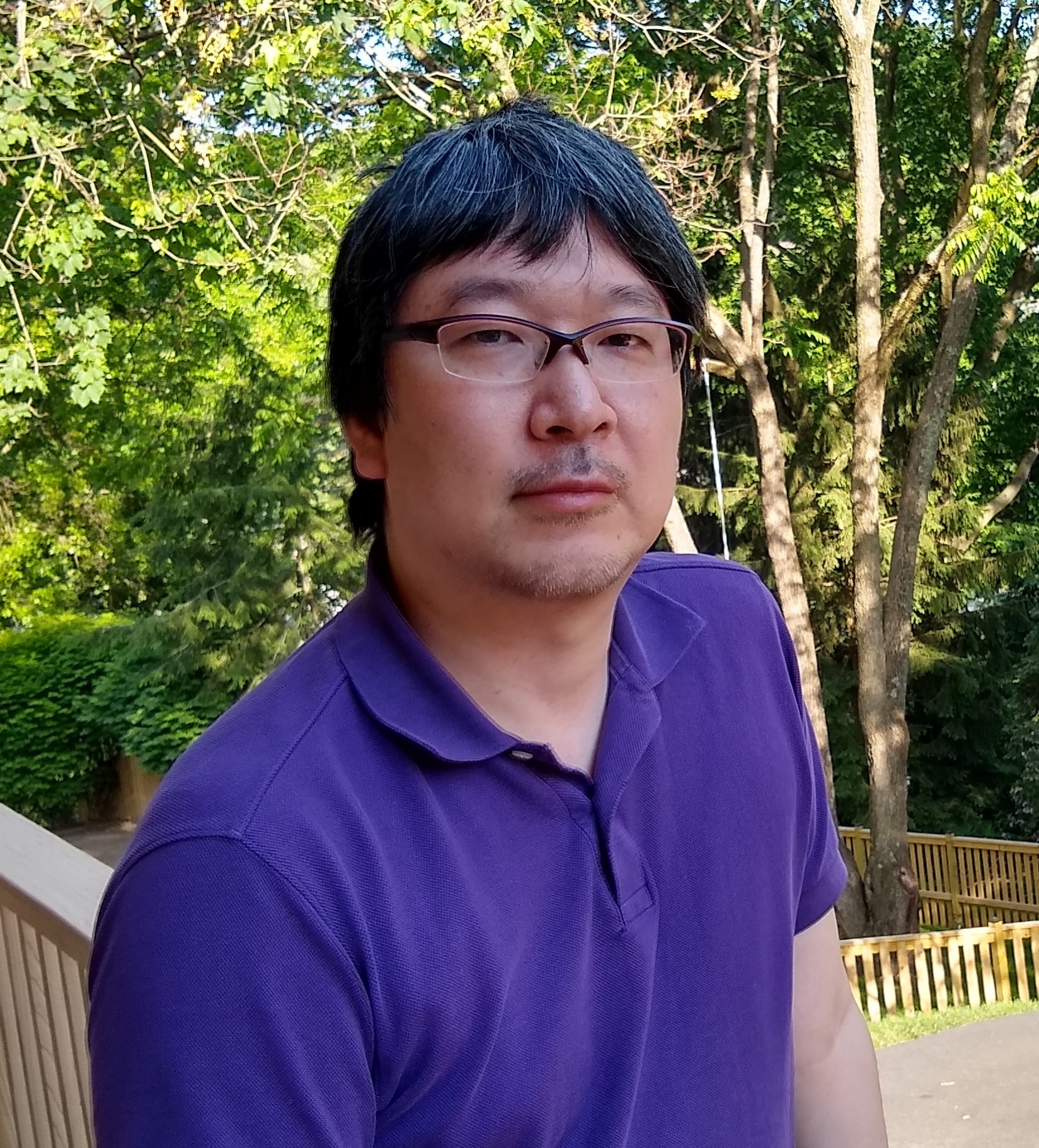
Abstract:
Speech foundation models are an active research area with the potential to consolidate various speech-processing tasks within a single model. A notable trend in this domain involves scaling up data volume, model size, and the range of tasks. This scaling trajectory has brought about significant changes in our research landscape, particularly regarding resource allocation. Notably, it has led to a division of research roles, where large tech companies primarily focus on building foundational models, while smaller entities, including academic institutions and smaller companies, concentrate on refining and analyzing these models. While this division has streamlined research efforts, there is a growing concern about the potential loss of explainability in these foundational models. This is primarily due to the limited transparency in the model-building process, often dictated by company policies. To address this concern, our group has started the development of large-scale speech foundation models. Our talk introduces Open Whisper-style Speech Models (OWSM), a series of speech foundation models developed at Carnegie Mellon University, reproducing OpenAI Whisper-style training using publicly available data and our open-source toolkit ESPnet. Crucially, our models exhibit several explainable behaviors thanks to the transparency inherent in our model-building process. In addition to showcasing the OWSM models, we discuss the related research efforts encompassing software development, data collection, cleaning, and model evaluation. Throughout this presentation, we would like to discuss how to address the research challenges posed by this shifting landscape within our speech and audio community.
Bio:
Shinji Watanabe is an Associate Professor at Carnegie Mellon University, Pittsburgh, PA. He received his B.S., M.S., and Ph.D. (Dr. Eng.) degrees from Waseda University, Tokyo, Japan. He was a research scientist at NTT Communication Science Laboratories, Kyoto, Japan, from 2001 to 2011, a visiting scholar at Georgia Institute of Technology, Atlanta, GA, in 2009, and a senior principal research scientist at Mitsubishi Electric Research Laboratories (MERL), Cambridge, MA USA from 2012 to 2017. Before Carnegie Mellon University, he was an associate research professor at Johns Hopkins University, Baltimore, MD, USA, from 2017 to 2020. His research interests include automatic speech recognition, speech enhancement, spoken language understanding, and machine learning for speech and language processing. He has published over 400 papers in peer-reviewed journals and conferences and received several awards, including the best paper award from the IEEE ASRU in 2019. He is a Senior Area Editor of the IEEE Transactions on Audio Speech and Language Processing. He was/has been a member of several technical committees, including the APSIPA Speech, Language, and Audio Technical Committee (SLA), IEEE Signal Processing Society Speech and Language Technical Committee (SLTC), and Machine Learning for Signal Processing Technical Committee (MLSP). He is an IEEE and ISCA Fellow.
Invited Talks:
Shusaku Egami (AIST AI Research Center)
"Event-Centric Knowledge Graph Construction and Its Applications"
Abstract:
Knowledge graphs typically represent static relationships between entities, such as thesaurus and human relationships. In contrast, Event-centric knowledge graphs, representing 5W1H and spatiotemporal changes of events, facilitate knowledge utilization in various environments, such as cyber-physical systems and embodied AI. This talk will introduce our recent works on event-centric knowledge graph construction and their applications, such as knowledge graph embedding and reasoning.
Bio:
Shusaku Egami received his PhD degree in engineering from The University of Electro-Communications in 2019. He is currently a senior researcher at the National Institute of Advanced Industrial Science and Technology, Japan. He is also a collaborative associate professor at The University of Electro-Communications. His research interests include semantic web, ontology, and knowledge graph.
Takato Horii (Osaka University)
"Emotion as emergent symbols from physical and social interactions"
Abstract:
In the fields of robotics, especially in the area of human-robot interaction, research on emotion recognition and expression has been widely conducted with the aim of achieving emotional communication with humans. However, are the "emotions" that these systems treat the same as those we experience in our daily lives? For robots to understand emotions and realize flexible communication with humans, it is essential to understand not only the recognition and expression of emotions but also the process of emotion generation.
In this talk, I introduce our attempt to understand the theory of constructed emotion from the perspective of 'symbol emergence in robotics,' which focuses on physical and social interactions both within and external to the agents' bodies. I will discuss the differentiation and structuring of emotions during human development, the formation of a conceptual space centered on human physical reactions, and the construction of emotional concepts through social interactions.
Bio:
Takato Horii is an Associate Professor at Osaka University. He received a Ph.D degree in engineering from Osaka University, Osaka, Japan, in 2018. His research interests include robot learning, modeling human cognition, and human-robot interaction.
Keisuke Imoto (Doshisha University)
"x-to-audio: General Audio Synthesis From Various Input Prompt"
Abstract:
The generative AI can be regarded as a conversion system between an
input prompt and system output. In the field of audio processing,
text-to-speech synthesis and score-to-music generation have been studied
in the literature. Recently, an "x-to-audio" system such as
text-to-audio and onoma-to-audio has been developed to generate more
general sounds including environmental sounds and foley sounds, however
the detailed investigation for the input prompts has not been fully
explored. In this talk, I will overview the recent achievements of the
x-to-audio system and discuss how the input prompts for an x-to-audio
system should be designed, as well as the challenges and possible future
research directions of the x-to-audio system.
Bio:
Keisuke Imoto is an associate professor at Faculty of Culture and
Information Science, Doshisha University and directs the Multimedia
Computing Laboratories. He has been engaged in research on sound
detection and acoustic scene analysis, anomalous sound detection,
general audio synthesis, and microphone array signal processing. He
served as a member of the organizing committee of DCASE Workshop (2020,
2023-2024) and a organizer of DCASE Challenge (2020-2024). He is a
senior member of the IEEE Signal Processing Society and a member of the
Acoustical Society of Japan (ASJ). He received the Awaya Award from ASJ
in 2013, the TAF Telecom System Technology Award in 2018, and the Sato
Prize ASJ Paper Award from ASJ in 2020.
Hiroshi Kera (Chiba University)
"Interplay of machine learning and algebraic computation."
Abstract:
Computational algebra has developed various algorithms for processing polynomials, including polynomial system solving, or more generally, Gröbner basis computation of an associated ideal. This talk introduces a machine learning approach circumventing explicit mathematical algorithm design. The machine learning models obtain solutions in near-constant time, offering a pragmatic compromise when mathematical methods timeout on large-scale instances. Interestingly, learning such algebraic computations also reveals new algebraic problems. This suggests an intriguing interplay between machine learning and the latest frontiers of computational algebra.
Bio:
Hiroshi Kera is an assistant professor at Chiba University. He received his Ph.D. at the University of Tokyo in 2020. He is working on the interdisciplinary domain of computational algebra and machine learning. He is also working in the computer vision domain, particularly on robust classification and recognition.
Hongyuan Mei (Toyota Technological Institute at Chicago)
"Towards Flexible Reasoning with Large Language Models as Informal Logic"
Programs
Abstract:
Formal logic programs are useful tools in artificial intelligence. However,
they require users to first express the problem in a formal logic language,
which is difficult to do for many real-world problems. In this talk, I will
discuss an alternative paradigm, using large language models (LLMs) as
informal logic programs. In this paradigm, the propositions are expressed
in natural language and the reasoning steps are carried out by a prompted
LLM.
This talk will present three problems effectively addressed by this
paradigm. The first is event sequence modeling and prediction, the task of
reasoning about future events given the past. The second is natural
language entailment, the task of determining whether a statement is
entailed by natural language premises. The third is embodied reasoning, in
which a robot needs to plan multiple steps to complete a task. For all
these problems, our paradigm achieves stronger results than classical
methods using formal logic programs and/or using LLMs as standalone
solvers. I will sketch a few future research directions, including
understanding how maximum-likelihood training of an LLM yields emergent
reasoning capabilities.
Bio:
Dr. Hongyuan Mei is currently a Research Assistant Professor at Toyota
Technological Institute at Chicago (TTIC). He obtained his PhD from the
Department of Computer Science at Johns Hopkins University (JHU), where he
was advised by Jason Eisner. Hongyuan's research spans machine learning and
natural language processing. Currently, he is most interested in harnessing
and improving the reasoning capabilities of large language models to solve
challenging problems such as event prediction. His research has been
supported by a Bloomberg Data Science PhD Fellowship, the 2020 JHU Jelinek
Memorial Award, and research gifts from Adobe and Ant Group. His technical
innovations have been integrated into real-world products such as Alipay,
the world's largest mobile digital payment platform, which serves more than
one billion users. His research has been covered by Fortune Magazine and
Tech At Bloomberg.
Eita Nakamura (Kyushu University)
"Recent developments and open problems in audio-to-score music transcription"
Abstract:
Music transcription is a task of converting a music audio signal into a
symbolic musical score; it is a fundamental technology for music
analysis and information processing. While the task is apparently
similar to speech recognition, there are various challenges unique to
music due to its complex data structure and diverse possibilities of
expression. In this talk, I discuss how deep learning approaches have
advanced the state-of-the-art performances in recent years and how
generative process modeling has been applied to complement the
limitations of end-to-end methods. I also argue the difficulty of
realizing a perfect music transcription system for general music and
suggest the need for cultural and cognitive considerations for further
research.
Bio:
Eita Nakamura is an Associate Professor in the Intelligence and Cultural
Evolution Laboratory at the Graduate School of Information Science and
Electrical Engineering, Kyushu University. He received his Ph.D. degree
in physics from the University of Tokyo in 2012. He worked as a postdoc
researcher at the National Institute of Informatics in Japan, Meiji
University, and Kyoto University. From 2019 to 2024, he was an Assistant
Professor at the Hakubi Center for Advanced Research, Kyoto University.
His fields of expertise include intelligence informatics and
interdisciplinary physics, with particular interests in mathematical
modeling of intelligent behaviors and evolutionary phenomena regarding
arts and creative cultures.
Erhan Oztop (Osaka University)
"Emergent Mirror and Imitation Mechanisms"
Abstract:
This presentation will bridge early mirror neuron modeling studies with recent end-to-end learning methods that can discover latent representations with interesting invariance properties. The modeling results will then be discussed from a (biological) neural reuse point of view.
Bio:
Erhan Oztop earned his Ph.D. at the University of Southern California in 2002. In the same year he joined the Computational Neuroscience Laboratories at the Advanced Telecommunications Research Institute International, (ATR) in Japan. There he worked as a researcher and later a senior research and group leader where he also served as vice department head for two research groups. Currently, he is a specially appointed professor in Osaka University at the Symbiotic Intelligent Systems Research Center (SISReC), Institute for Open and Transdisciplinary Research Initiatives (OTRI) and also a professor at the Computer Science Department of Ozyegin University. His research interests include cognitive and developmental robotics, human-in-the loop robotic systems, computational neuroscience and artificial intelligence.
Jun Sakuma (Tokyo Institute of Technology)
"AI Security: a new perspective of defensive attack"
Abstract:
Deep neural networks are useful in various recognition and decision-making tasks. On the other hand, deep neural networks are known to be at risk of having their behaviour manipulated by adversarial attacks, and research on understanding its vulnerability and establishing defending methodologies have attracted considerable attention. The talk will examine how the diverse forms of deep learning usage, along with the expansion of deep learning application areas, have affected the attack methodologies of deep learning. We also discuss methodologies that utilise deep learning attack techniques for defence as a new direction for deep learning defence.
Bio:
Jun Sakuma received a Ph.D. in Engineering from the Tokyo Institute of Technology, Tokyo, Japan, in 2003. He has been a professor at the School of Computing at Tokyo Institute of Technology since 2023. He has also led the Artificial Intelligence Security and Privacy team in the Center for Advanced Intelligence Project, RIKEN, since 2016. His work focuses specifically on AI security and responsible AI. He serves as area chair, senior meta reviewer, and reviewer of top conferences in AI and security, including AAAI, IJCAI, NeurIPS, ICML, ICLR, and Usenix Security. His research work on AI fairness, published in ECML/PKDD2012, was awarded as the test of time award of ECML/PKDD2022.
Yoko Yamakata (The University of Tokyo)
"Food AI for Human and Planet Health"
Abstract:
We eat more than eighty thousand times during our lifetime. Whether we
like it or not, no one can escape this routine. Delicious meals please
us, but if we make the wrong choices, they can also cause serious
health problems, such as obesity and diabetes. In addition, food is a
consumption activity, and its production and delivery require many of
the earth's resources. In recent years, abnormal weather has caused
frequent droughts and floods worldwide, and the issue of sustainable
food has emerged. What can food AI do to deal with these issues?
This keynote introduces food AI technologies. First, let us focus on
human health. We are developing RecipeLog and FoodLog Athl, smartphone
applications that allow users to upload a photo of a meal, identify
the name of the meal through image recognition, calculate nutritional
values such as energy, protein, fat, and carbohydrates based on the
recipe, and create a food log. Traditionally, dietary management for
patients and athletes involved manual analysis of nutritional intake
by dietitians based on their manual food records, but under and
over-reporting, in addition to high human costs for analysis, has been
problematic. Our experiments have shown that information obtained by
image recognition of food photos is less costly in terms of human
labor and more accurate than manual recording.
The latter part of the talk will introduce food AI for the planet's
health. In the process of producing food, earth resources are mined to
obtain phosphate fertilizer and to extract fuel for breeding and
transportation. By calculating the amount of this mining for each food
ingredient, it was estimated that, for example, an average of 5.2 kg
of ground is mined to provide one serving of beef stew. Our goal is to
visualize these facts in a way accessible to ordinary people and to
change their behavior in making food choices better for all.
Bio:
Professor Yoko Yamakata received her Ph.D. from the Graduate School
of Informatics, Kyoto University, in 2007. She served as a lecturer
and later as an associate professor at Kyoto University for six years
starting in 2010. In 2015, she became a JSPS Research Fellow and
conducted research as a visiting scholar at the University of Sussex
in the UK, accompanied by her two sons. In 2019, she was appointed as
an Associate Professor at the Graduate School of Information Science
and Technology, the University of Tokyo, and in 2024, she became a
Professor at the Information Technology Center of the University of
Tokyo. Her specialty is in multimedia information processing,
primarily focusing on deep learning techniques for text and images.
She has a keen interest in AI-based technologies supporting food
applications.
Natsue Yoshimura (Tokyo Institute of Technology)
"Simple genetic algorithm for on-site system optimization: A communication system for individuals with completely locked-in syndrome."
Abstract:
Brain activity signals are the only available biological signal for communication systems for patients with completely locked-in syndrome, in which all voluntary movement functions, including the eyes, are impaired due to the progression of amyotrophic lateral sclerosis (ALS). While the possibility has emerged that the future may offer a choice between an invasive method in which electrodes are implanted in the brain or a non-invasive method in which measurements can be taken from outside the skull, this presentation will illustrate an effort to accommodate users who currently require means of communicating their intentions. Using electroencephalography (EEG), this system targets the discrimination between "yes" and "no" responses, an aspect of communication that is fundamental but challenging to identify as a feature of brain activity, and adopts an approach to "generate" brain regions with the distinctive traits of the responses. In addition, the talk will introduce the effectiveness of employing a genetic algorithm to select optimal sensor positions for a specific day by showcasing a real-life case study. This approach is expected to provide on-site adjustments for systems, which are intended to benefit users in practical application, and to address the issue of EEG sensor position fluctuations that affect intention extraction on a daily basis.
Bio:
Natsue Yoshimura is a Professor at Tokyo Institute of Technology. Her research interests include brain machine/computer interfaces, brain activity information decoding relating to motor control, speech, and emotion, using noninvasive brain activity recording methods, such as electroencephalography and functional magnetic resonance imaging. She completed her Ph.D. at the University of Electro-Communications in 2009 and M.S. at Tokyo Medical and Dental University in 2006, after working for several industries.